What advancements are being made in natural language processing and understanding?
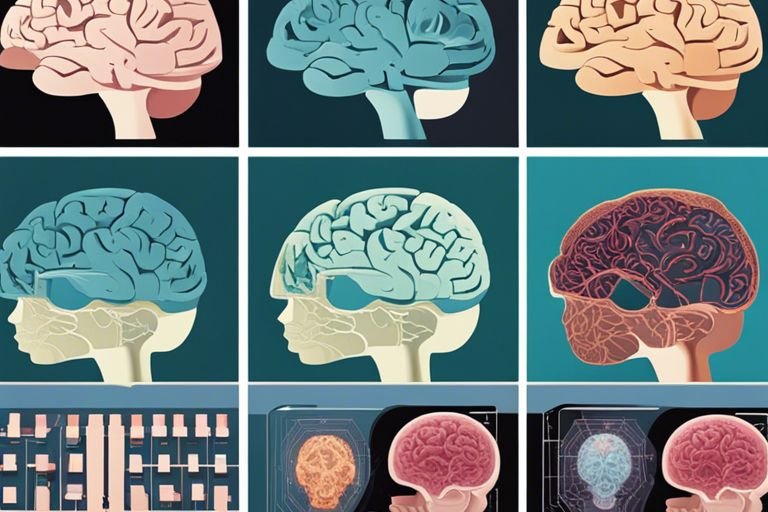
Salutations, curious reader! As you delve into the realm of natural language processing and understanding, it is paramount that you stay informed about the remarkable advancements being made in this field. From cutting-edge algorithms to the utilization of deep learning techniques, researchers and developers are consistently pushing the boundaries of language comprehension and analysis. These breakthroughs are not only revolutionizing the way machines interpret human language, but also enhancing communication between individuals and technology. However, it is important to remain cautious of the ethical implications and potential misuse of these advancements, as manipulation of natural language processing could pose significant dangers if left unchecked.
Key Takeaways:
- Enhanced language model development: Advancements in natural language processing have led to the creation of more sophisticated language models, such as GPT-3, that can generate human-like text and understand and respond to a wide range of queries.
- Improved sentiment analysis: Natural language processing tools now have the ability to accurately identify emotions and opinions within text, aiding in sentiment analysis for companies seeking to understand customer feedback and social media chatter.
- Multi-language understanding: There is progress in the development of natural language processing models that can understand and process multiple languages, enabling more effective communication and understanding across different cultures and regions.
Evolution of NLP Technologies
Clearly, the field of natural language processing (NLP) has seen significant advancements over the years. As technology continues to progress, the evolution of NLP technologies has paved the way for more advanced and efficient language processing and understanding capabilities.
From Rule-Based to Machine Learning Approaches
Historically, NLP technologies relied heavily on rule-based approaches, where language processing systems were programmed with a set of rules to identify and understand language patterns. While effective to some extent, these rule-based systems often lacked the flexibility and adaptability needed to handle the intricacies of natural language. However, with the advancements in machine learning, NLP has shifted towards more dynamic and adaptive approaches. By leveraging machine learning algorithms, NLP systems can now learn from data, adapt to new language patterns, and continuously improve their understanding of natural language. This evolution has vastly improved the accuracy and efficiency of NLP technologies, allowing for more nuanced and context-aware language processing.
Breakthroughs in Deep Learning and NLP
One of the most significant breakthroughs in NLP has been the integration of deep learning techniques. Deep learning, a subfield of machine learning, involves the use of neural networks to process and understand complex data inputs. When applied to NLP, deep learning models have revolutionized language processing capabilities. You can now use deep learning to train NLP models to interpret and generate human-like text, enabling more robust and natural language understanding. This has led to the development of advanced language processing applications, such as sentiment analysis, language translation, and chatbots. The ability of deep learning models to analyze and interpret language at a deeper level has unlocked new possibilities for NLP, empowering you to interact with and understand language in a more human-like manner.
Current Trends in NLP
One of the most exciting aspects of natural language processing (NLP) is the rapid pace of advancement in the field. As NLP continues to evolve, several key trends have emerged that are shaping the future of the technology. In this chapter, we will explore some of the current trends in NLP that are driving progress and innovation in the field.
Pre-trained Language Models
Pre-trained language models have emerged as a game-changer in NLP, allowing for more efficient and accurate natural language understanding. These models are trained on vast amounts of text data, enabling them to learn the nuances of language and context. By leveraging pre-trained language models, you can significantly improve the performance of your NLP applications without the need to start from scratch. The ability to fine-tune these models for specific tasks or domains can significantly enhance the user experience and the overall effectiveness of NLP applications. However, it is important to be cautious of the potential biases and ethical implications that may arise from using pre-trained language models, as they can inherit and perpetuate harmful stereotypes and misinformation.
Multimodal NLP and Transfer Learning
Multimodal NLP and transfer learning have emerged as key trends in NLP, allowing for more comprehensive and efficient natural language processing across different modalities and domains. This approach enables you to leverage knowledge and insights from one task or domain to enhance the performance of another. By incorporating multiple modalities such as text, images, and audio, you can gain a deeper understanding of the context and meaning behind the language. Transfer learning also allows you to transfer knowledge from one task or dataset to another, enabling more efficient learning and utilization of resources. While these advancements have the potential to revolutionize NLP, it is important to carefully consider the ethical implications and potential biases that may arise from transferring knowledge across different domains and modalities.
Applications and Real-World Impacts
Not only is natural language processing and understanding advancing at a rapid pace, but the real-world impacts and applications of these advancements are also growing. In this section, we will explore the various ways in which NLP is being applied and its effects on different aspects of our lives.
NLP in Healthcare
One of the most significant applications of natural language processing in healthcare is in the analysis of medical records and clinical notes. By using NLP, healthcare providers can extract crucial information from these unstructured data sources to make better-informed decisions regarding patient care. For example, NLP can help in identifying patterns in patient data to predict disease outbreaks, improve diagnostic accuracy, and personalize treatment plans. Your healthcare provider may use NLP-powered tools to analyze your medical history, ensuring that you receive the most effective and personalized care based on your unique health data.
Ethical Considerations and Mitigating Bias
It is important to consider the ethical implications of the widespread use of NLP and take steps to mitigate bias. While NLP has the potential to revolutionize various industries, it also holds the risk of perpetuating biases present in the input data. By recognizing and addressing biases, you can ensure that NLP technologies are fair and inclusive. Whether it’s in hiring processes, predictive policing, or financial decision-making, you have the power to advocate for responsible use of NLP and ensure that these technologies do not reinforce societal inequalities.
Future Directions
For future directions in natural language processing and understanding, the focus is on advancing machine understanding, addressing challenges, and finding potential solutions to improve the overall efficiency and accuracy of NLP systems.
Advancements in Machine Understanding
With the rapid development of NLP technology, advancements in machine understanding have become a key area of focus. The goal is to enable machines to not only process and analyze language but also to comprehend and interpret the underlying meaning and context. This involves incorporating more advanced algorithms, such as deep learning and neural networks, to improve the ability of machines to understand and respond to natural language input. This advancement is critical for enhancing the effectiveness of chatbots, virtual assistants, and other NLP applications.
Challenges and Potential Solutions
Despite the progress in NLP, there are still significant challenges that need to be addressed. One of the primary challenges is the ambiguity and complexity of natural language, which can lead to errors and misunderstandings in NLP systems. Improving the ability of machines to handle ambiguity and context in language is crucial for ensuring accurate and reliable results. Potential solutions involve leveraging context and contextual information, implementing advanced language models, and refining algorithms to better handle ambiguous language constructs. Additionally, there is a need to address ethical and privacy concerns surrounding the use of NLP technology, which requires careful consideration and implementation of safeguards to protect user data and ensure fair and unbiased processing of language data.
Advancements in Natural Language Processing and Understanding
Conclusively, you can expect to see significant advancements in natural language processing and understanding in the near future. Companies and researchers are constantly pushing the boundaries of what is possible in this field, with developments in machine learning, deep learning, and neural networks leading to more accurate and sophisticated language processing systems. As a result, you can anticipate improved language translation, voice recognition, and text analysis tools that will revolutionize how we interact with technology and communicate with one another. These advancements will not only enhance the efficiency and accuracy of language processing systems, but will also open up new possibilities for artificial intelligence and human-computer interaction.